AI Accelerator – Microsoft 365 Copilot
The key to any successful AI implementation is careful, meticulous preparedness of your data environment, technology infrastructure, and security posture.
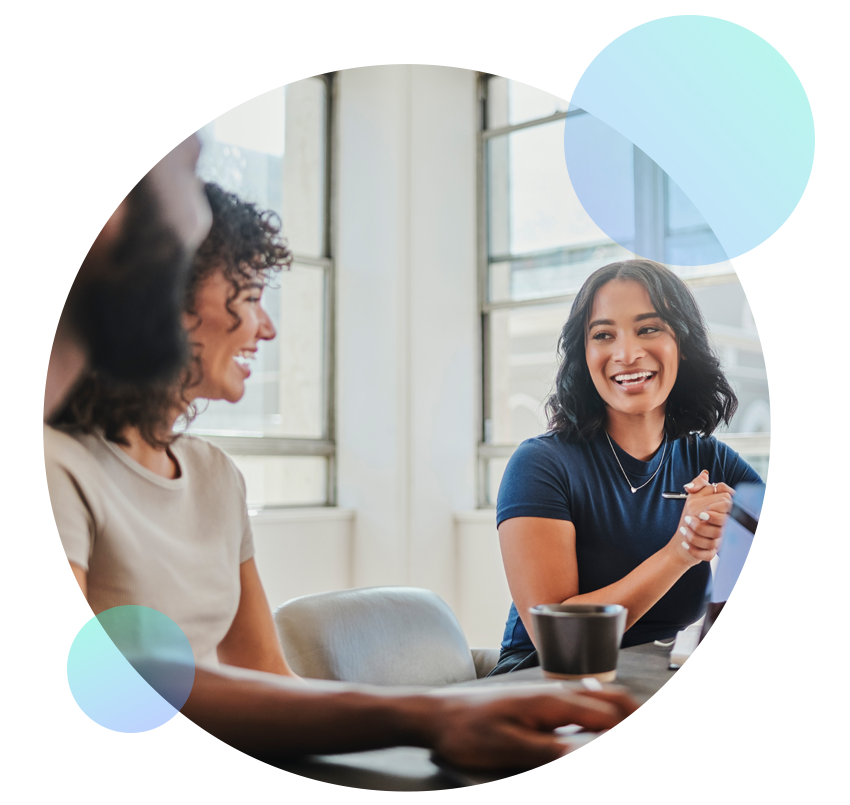
AI-driven future
Assess
An agnostic approach to determining the value of emerging technologies to enhance each client’s current environment and achieve desired business outcomes.
Design
A holistic approach to designing a personalized mix of interconnected cloud-native applications, platforms, and operations.
Deploy
Organizations can eliminate pitfalls and mitigate risk by partnering with a trusted advisor to migrate mission-critical applications and ensure success.
Operate
Our expert team monitors modernized applications and associated cloud and on-prem platforms to ensure peak performance.
Consulting and assessments
Building a technology modernization roadmap starts with assessing and documenting your current environment. Together, we outline recommendations for modernizing applications, platforms, and IT operations based on your business’s strategic priorities.
Consider adopting a DevOps best practices plan to promote a culture of business innovation and continuous process improvement.
Design and development
Modern digital technologies allow for the continuous deployment of new features, and cloud-native architecture enables better security, future-proofed scalability, performance, and high availability.
Our strategy involves re-writing, re-architecting, and re-platforming legacy apps into cloud-native applications with business outcomes at the forefront and security at the center. We aim to maximize performance and availability by running apps on proven platforms and across robust networks.
Secure migration
Workloads must be securely migrated from one data center or cloud environment to another with as little impact on production and in a secure and protected manner.
New application and platform environments are designed and built based on your business objectives and preferred consumption models (cloud, hybrid, on-prem). Modernized applications are tested thoroughly and migrated securely into production with little impact on day-to-day operations.
Operations and management
Managing rapidly evolving technology is a complex and challenging process. Businesses can simplify workflows and optimize staff productivity by maintaining and securing their technology environment with a CBTS managed services program.
Our team of experts monitors modernized applications and associated cloud
and on-prem platforms to ensure peak performance and availability. Updates
and patches are applied automatically to ensure ongoing compliance. Backup
and recovery services and continuous security monitoring provide powerful data
protection.
CBTS solutions enable organizational
transformation and deliver meaningful
business outcomes
Application services
A suite of solutions designed to help companies modernize, migrate, and manage their app portfolios.
Cloud
Cloud-based tools and solutions for infrastructure, networking, security, applications, and communications.
Consulting
Suite of advisory and assessment services that help guide an organization’s technology roadmap.
Digital workplace
Modern workplace solutions that optimize employee collaboration, improve productivity, and lead to exceptional customer service.
Infrastructure
Core technology platforms built to optimize resilience, security, and operational efficiency.
Managed services
Offload IT burden with our certified expertise and longstanding technology partnerships.
Security
Robust and mature security solutions effective for identifying and mitigating risk.
Challenges
Solutions
Outcomes
Over time, technology ages, software becomes outdated, and vulnerable, legacy systems crawl as critical issues negatively impact revenue.
%
of businesses are not harnessing the full potential of digital technologies.
McKinsey & Company
%
of businesses experience delays in migration projects by one quarter or more.
McKinsey & Company
%
of companies have experienced a data compromise or breach within the past 12 months.
Dell
From consulting to design and implementation to ongoing management and optimization, our expert professionals have the deep technology experience to transform your technology environment into a growth engine for your organization.
%
of companies see cloud technology as essential for growth.
Deloitte
%
of board directors say digital transformation is embedded in all business growth strategies.
Gartner
%
of senior executives say digital transformation is an organizational priority.
Gartner
By modernizing infrastructure, migrating apps to the Cloud, and mitigating security risks, businesses can achieve outcomes that drive significant growth.
%
reduction in IT spend after adopting serverless computing.
AWS
%
increase in organization revenue for highly modernized organizations.
AWS
%
faster time to resolve security incidents after adopting managed data.
AWS
Case studies
View All

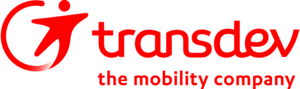
First Transit, Inc., now a part of TransDev, is North America’s largest private-sector provider of mobility solutions, moving more than 300 million passengers annually. The company provides consulting, management, and operations for more than 300 locations in 41 states, Puerto Rico, and four Canadian provinces. Its clients include transit authorities, states, municipalities, universities, and private companies.
Problem
First Transit needed to generate custom reports to support business objectives without using expensive developers and contractors. The client wanted to empower business users to create apps to access data and reports on demand without involving IT.
CBTS solution
CBTS designed a secure Microsoft Power Platform Framework to make app development easier, ensure governance, and empower “citizen developers.” CBTS established proper controls and a center of excellence, allowing no-code/low-code development to obtain data and reports without risking security.
Outcome
First Transit has the framework, processes, and policies to enable Power Platform application development. Updated security, monitoring, alerts, and automated policies allow the client to drive platform adoption without being restrictive.
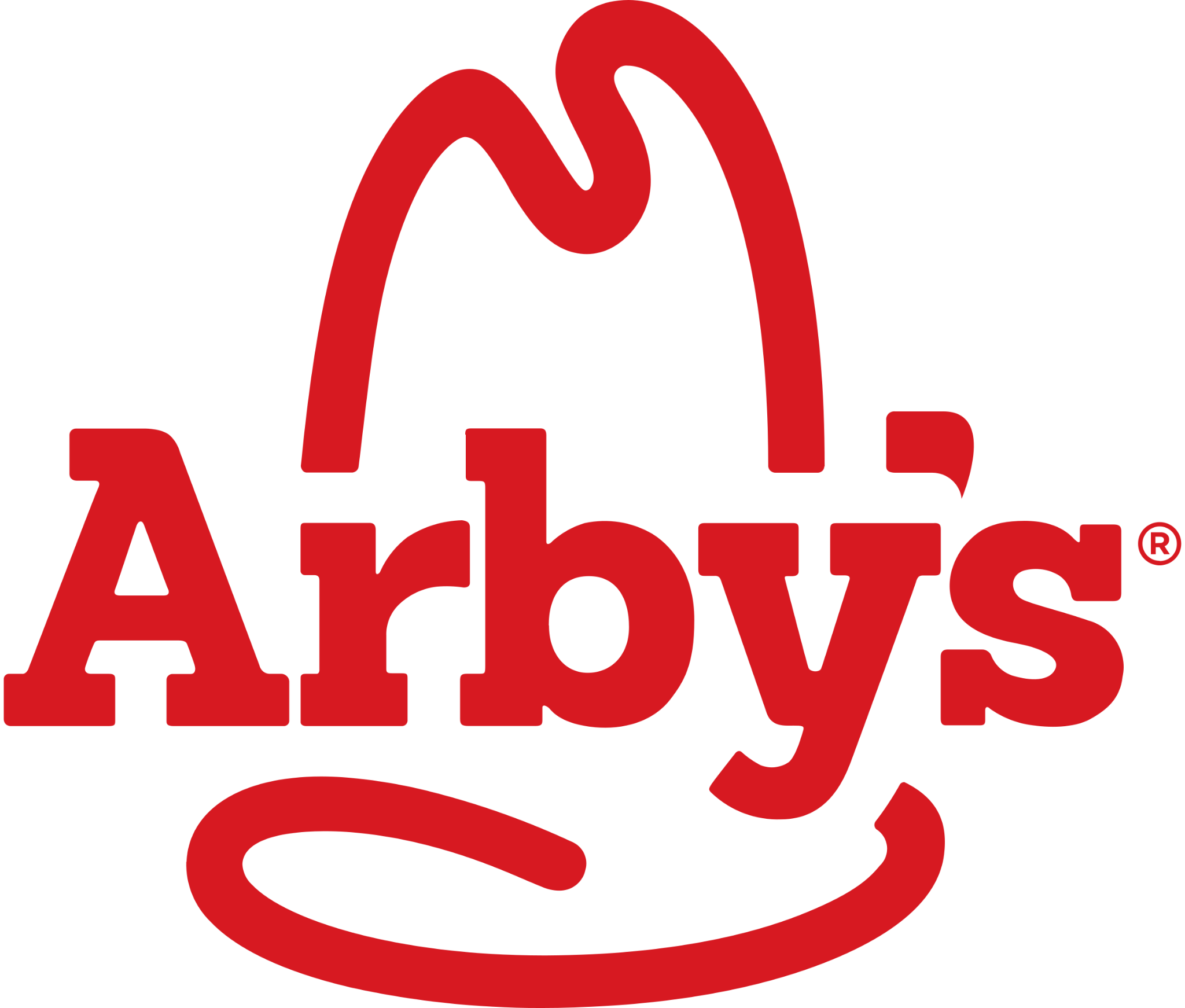
Restaurant Management Inc. (DBA Arby’s)—a franchisee of the nation’s second-largest quick-service sandwich chain—needed to enhance its technology to stay relevant with its customer base.
Problem
Restaurant Management Inc. (DBA Arby’s) needed to upgrade its outdated cloud environment to meet licensing requirements. However, pandemic-related microchip shortages created global obstacles for innovation. Obsolete technology and a complex network of service providers further hindered efforts to improve customer service.
CBTS solution
CBTS swiftly deployed a tailored NaaS solution using Cisco Meraki, implementing it across 67 client locations and 138 devices. The CBTS team collaborated closely with Cisco to expedite the cloud migration process and find suitable hardware alternatives. CBTS demonstrated agility by successfully deploying distinct NaaS solutions across two different POS systems for the client.
Outcome
The outcome was an efficient, managed network that enabled Restaurant Management Inc. (DBA Arby’s) to prioritize enhancing the customer experience. This scalable network could easily accommodate new locations and reconfigurations. Implementing a robust cloud network solution improved store efficiency for the client. By streamlining the network of managed services, the client could dedicate more attention to improving the customer experience.
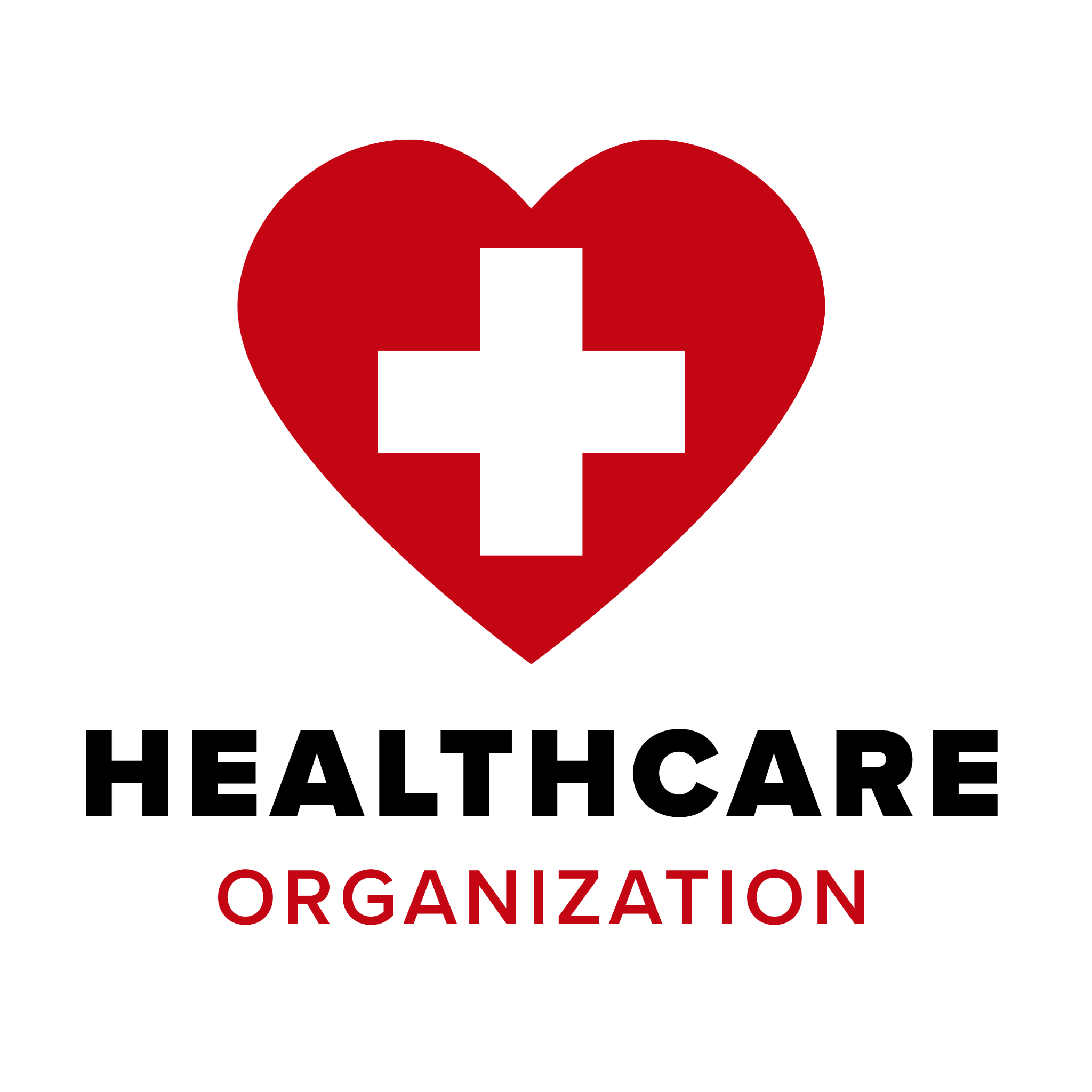
The client is a large, complex healthcare organization navigating transformational change due to ongoing consolidation in the industry.
Problem
The client's IT department faced challenges with aging and disparate infrastructure. Scaling opportunities were limited, leading to downtime for critical applications. Due to the organization's infrastructure issues, they could not focus on value-added digital initiatives for improving patient care.
CBTS solution
The client engaged CBTS Infrastructure to analyze its environment and create a phased roadmap to support organizational goals. A deep dive identified pain points and future IT needs. Recommendations included high-performance computing storage, a converged infrastructure (CI) solution based on FlexPod, automation with VMware vCloud Suite, and Disaster Recovery as a Service. CBTS then implemented the project, addressing each client issue and providing the foundation for future growth and innovation by freeing IT resources with remote monitoring and management.
Outcome
CBTS Infrastructure became a trusted advisor to the client's CIO, delivering a validated business case identifying benefits and risks for infrastructure improvements and increased bandwidth to support business and patient care initiatives.
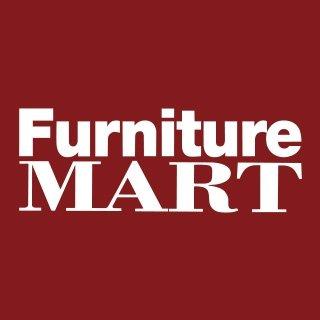
The Furniture Mart is a top 40 furniture retailer with more than 50 locations, rapidly expanding across the Midwestern United States. Priding itself on beautiful, affordable furniture and sleep solutions, the company aims to create extraordinary home lives for a wide range of customers.
Problem
The Furniture Mart wanted to leverage modern technology to optimize customer experience. Additionally, the company found it challenging to ensure clear communication between its corporate headquarters and numerous retail outlets.
CBTS solution
CBTS designed the omni-channel system with customers in mind, enabling them to interface with The Furniture Mart via their preferred communication pathway (e.g., voice, SMS text, chat, or e-mail). CBTS delivered contact center advances through its Five9 CCaaS platform, which combines Five9 software with trusted Cisco UCaaS solutions. The Five9 blended contact center unifies inbound and outbound calling features so users can access multiple applications simultaneously from a single cloud-based platform.
Outcome
With its new omni-channel environment, The Furniture Mart has merged UCaaS and CCaaS into a single solution. These services working in unison allow the company to pass calls seamlessly from the contact center to a knowledgeable staff member in logistics, shipping, scheduling, or any other department. With CBTS solutions in place, the client decreased risk due to aging communications infrastructure and reduced costs and maintenance burden. Ultimately, a customer-centric network enables the client to meet customer demands rapidly in a crowded marketplace.
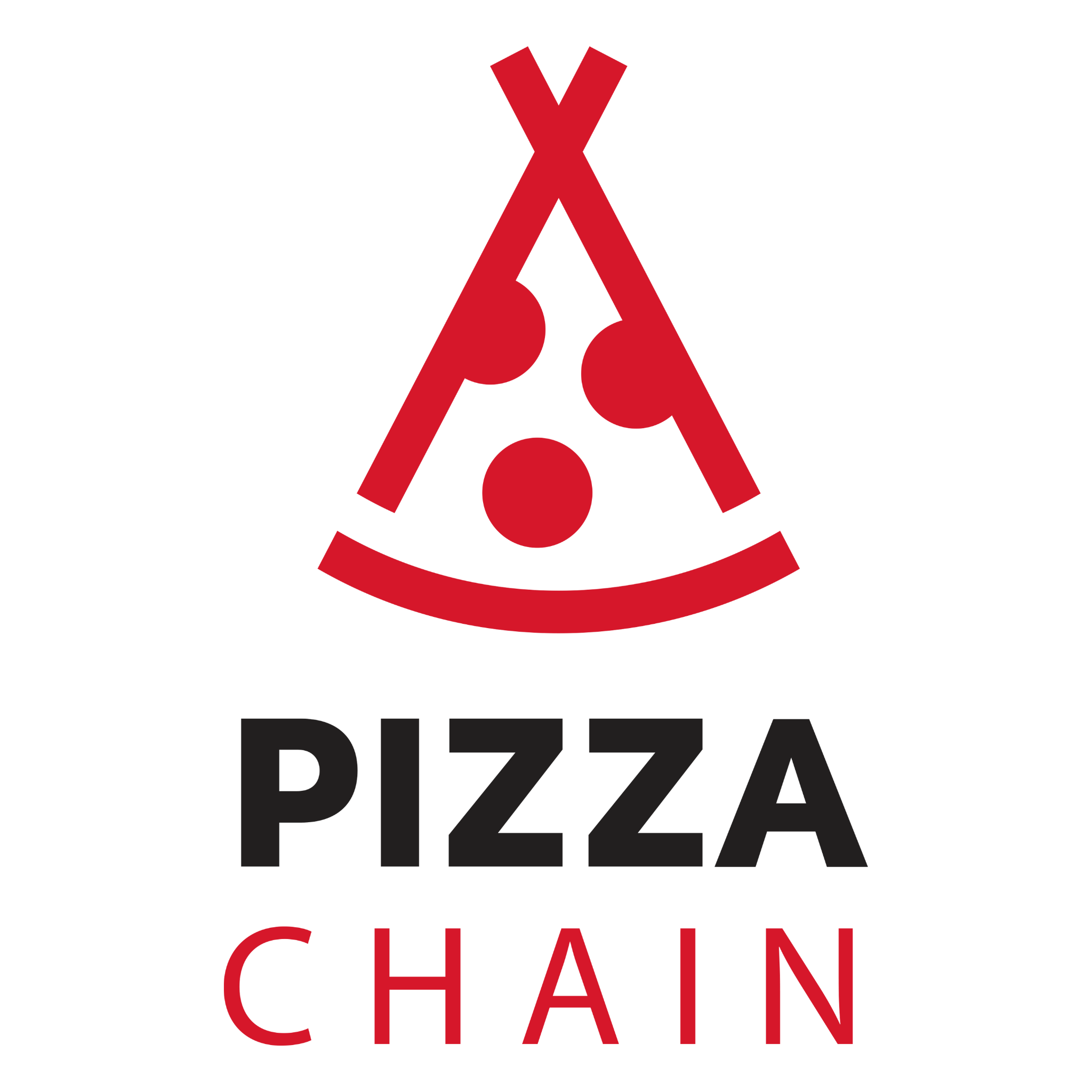
The client is a leader in the "fast-casual pizza" business, with $166 million in annual sales and 153 units in seven states. Additionally, this pizza chain operates over 100 "take-and-bake" kiosks in grocery stores and offers products at 18 entertainment and sports venues.
Problem
The company was continuously making improvements to its pizza ordering system as a strategic focus area to improve and streamline customer experience. With web and mobile options available for pizza ordering, the company wanted to reinforce and enhance its infrastructure to make it even easier to order online.
CBTS solution
CBTS Managed Services specialists, engineers, technical services, and operations professionals collaborated with the client's IT team to provide the framework to support the company's initiatives. Within ten days, CBTS provided:
• Web hosting service as part of the CBTS IaaS solution.
• Internet accessibility at all store locations through VPN tunnels.
• Infrastructure monitoring and management of firewalls, servers, VDOMs, and VPNs.
Outcome
After launch, the client enjoyed 33% savings on IT services, improved service delivery, and end-to-end services/support. Since deploying the IaaS solution, the client has engaged CBTS for additional services, including CBTS IP VPN solution for headquarters and franchise store operations, Avaya phone support, and hosted back office application management.
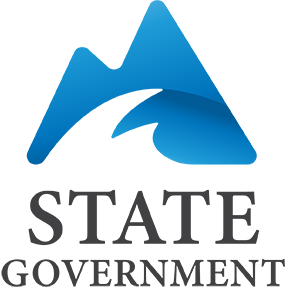
Our client is a large Midwestern state with 92 counties, 16 metropolitan areas, 117 incorporated cities, 450 towns, and several smaller statistical divisions and regions.
Problem
The state wanted to re-evaluate the effectiveness of its current SoI VaaS communication platform. The client already used Microsoft E3/5 and G3/5 licenses and had over 30,000 computer accounts using Microsoft Teams. However, they wanted to modernize the legacy SoI VaaS communication platform to Microsoft Teams using Direct Route for PSTN calling.
CBTS solution
CBTS assessed the environment and built a migration roadmap. Healthcare users stayed on the existing SoI VaaS Cisco platform for compliance purposes, and all other users migrated to Microsoft Teams Voice. After a 90-day trial in which CBTS delivered PSTN voice into the state's Microsoft Teams environment via Direct Route, the state moved forward with the migration.
Outcome
State employees can now work from any device or location using the Microsoft Teams application. This flexibility has dramatically improved productivity and collaboration within the state's geographically diverse footprint. In addition, the state has a go-to partner in CBTS to support its current dual-platform system.
Long-standing strategic partnerships with industry leaders
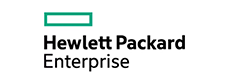
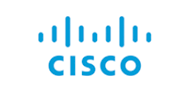
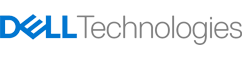
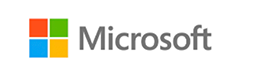
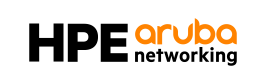
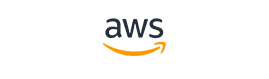
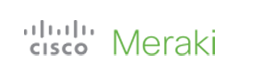
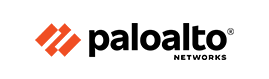
CBTS by the numbers
3,000+
2,400+
Dedicated employees
$1.3 B+
Annual revenue
2,500+
Technology certifications
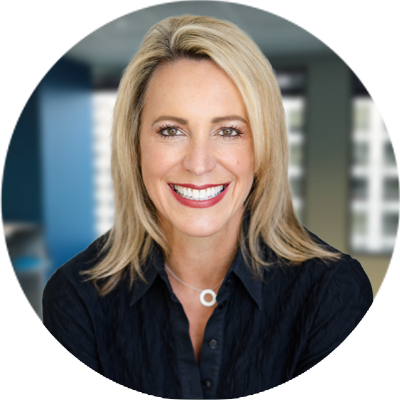
“Our clients rely on us for technology solutions that enable operational efficiencies and deliver transformational growth to their business. Our customer-first mindset and commitment to their success are at the heart of everything we do.”
– Kristin Russell, CEO, CBTS